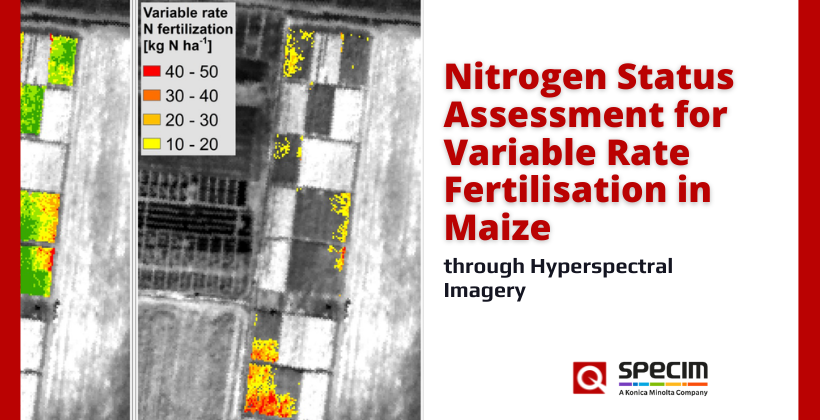
Remote Sens. 2014, 6(7), 6549-6565; https://doi.org/10.3390/rs6076549
Abstract
This paper presents a method for mapping the nitrogen (N) status in a maize field using hyperspectral remote sensing imagery. An airborne survey was conducted with an AISA Eagle hyperspectral sensor over an experimental farm where maize (Zea mays L.) was grown with two N fertilisation levels (0 and 100 kg N ha−1) in four replicates. Leaf and canopy field data were collected during the flight. The nitrogen (N) status has been estimated in this work based on the Nitrogen Nutrition Index (NNI), defined as the ratio between the leaf actual N concentration (%Na) of the crop and the minimum N content required for the maximum biomass production (critical N concentration (%Nc)) calculated through the dry mass at the time of the flight (Wflight). The inputs required to calculate the NNI (i.e., %Na and Wflight) have been estimated through regression analyses between field data and remotely sensed vegetation indices. MCARI/MTVI2 (Modified Chlorophyll Absorption Ratio Index/Modified Triangular Vegetation Index 2) showed the best performances in estimating the %Na (R2 = 0.59) and MTVI2 in estimating the Wflight (R2 = 0.80). The %Na and the Wflight were then mapped and used to compute the NNI map over the entire field. The NNI map agreed with the NNI estimated using field data through traditional destructive measurements (R2 = 0.70) confirming the potential of using remotely sensed indices to assess the crop N condition. Finally, a method to derive a pixel based variable rate N fertilisation map was proposed as the difference between the actual N content and the optimal N content. We think that the proposed operational methodology is promising for precision farming since it represents an innovative attempt to derive a variable rate N fertilisation map based on the actual crop N status from an aerial hyperspectral image.
1. Introduction
Nutrients and various chemicals are usually supplied to agricultural soils to improve the crop yield. Excessive use of fertilisers (including nitrogen, N) should be avoided to minimise environmental impacts. In fact, although N fertilisation improves plant development, the fate of the non-absorbed portion arouses concerns due to leaching phenomena and to the emissions in the atmosphere [1] of greenhouse gases such as dinitrogen monoxide (N2O). However, even if the use of N fertilisers can be reduced without significantly influence the final yield [2], farmers often administer N supplies in excess in order to avoid any N deficit and assure profits at the end of the season [3]. To avoid such an excessive fertilisation, one of the most important steps at European level was the Nitrate Directive (91/676/EEC) in 1991 concerning the protection of ground and surface waters against pollution caused by nitrates (NO3−) from agricultural sources. This directive imposed the identification of waters containing more than 50 mg L−1 of NO3− (or that could contain this concentration if no action is taken to reverse the trend), and the adoption of action programmes on these vulnerable areas. The last European Commission report on the implementation of this directive for the period 2008–2011 states that the consumption of chemical fertilisers is decreasing. A tool for the in-season detection of N deficient areas is needed in order to administer the fertilisers only where necessary. In this context, the concept of critical N concentration (%Nc) [4,5] was proposed as the minimum N concentration in shoots required to produce the maximum aerial biomass at a given time. The %Nc declines exponentially as a function of aboveground dry mass accumulation (W). Species-specific relationships between %Nc and W have been proposed as references to be compared with actual %Nc and W measurements to derive the crop-specific N needs at each growth stage. Nitrogen needs are formalised by means of the Nitrogen Nutrition Index (NNI) [6,7] defined as the ratio between the actual leaf N concentration (%Na) measured in the field at a specific growth stage and the %Nc predicted by the reference function %Nc = f(W). NNI values close to 1 indicate plants not limited by N availability, while values lower or higher than 1 indicate N deficiency or excessive fertilisation, respectively [6]. NNI is traditionally calculated through field measurements that are quite time consuming. As a result, a reduced number of plants is usually sampled and the spatial heterogeneity of N needs is poorly represented. The estimation of the NNI based on spectral data has been proven feasible using field spectrometers operated on the ground [8] or mounted on tractors [9]. However the possibility to detect the N status using remotely rather than proximal sensed information has still to be tested [10]. The precise and effective remotely sensed estimation of the NNI input parameters (i.e., %Na and W) would provide a cost-effective detailed spatial characterisation in order to produce maps of crop nutritional deficit. Several studies demonstrated that leaf chlorophyll concentration can be estimated through hyperspectral vegetation indices based on the visible and red edge (680–760 nm) spectral domains [11–15]. Hyperspectral sensors are characterised by a high number of narrow and contiguous acquisition bands that allow a better description of specific portions of the electromagnetic spectrum compared to broadband sensors and, thus, better performances in biochemical parameter estimation [16]. The correlation between leaf pigments and leaf N incorporated in chlorophyll molecular structure [17,18] justified the use of vegetation indices for the determination of plant N condition [19–24]. Moreover, hyperspectral data have been also successfully used to estimate aboveground biomass accumulation, the second input required for NNI computation, using combinations of visible and near infrared reflectance in the form of simple or normalised ratios [25,26]. Nevertheless, the use of remote sensing to monitor crops in precision farming is still limited although its high potentiality in providing spatially detailed information to support site-specific management. This approach would result in reduced economic costs for farmers and a reduced impact on environmental resources. In this study we used hyperspectral remote sensing imagery to estimate the N concentration and the dry mass on the basis of relationships with field data acquired during the flight.
An airborne campaign was conducted with an AISA Eagle (Specim, Oulu, Finland) hyperspectral sensor over an experimental maize field. The main goals were (i) to determine crop specific N needs from airborne data through the calculation of the NNI map and (ii) to quantify the N deficit or surplus in different areas with respect to a reference optimal N content to provide variable rate N fertilisation maps. We finally proposed an empirical method based on the comparison of the N content accumulated in the aboveground dry mass in each pixel with the one found in the pixels identified as in optimal conditions by the NNI map.
2. Materials and Methods
2.1. Experimental Design
The experiment was conducted at the Vittorio Tadini experimental farm (44°58′49.0″N, 9°40′48.50″E, elevation 87 m a.s.l.) located in Gariga di Podenzano (PC) in Northern Italy. Twenty-four maize (Zea mays L.) plots sized 15 m × 16.5 m were organised in four replicates (blocks), as depicted in Figure 1. In each block, two N fertilisation levels (i.e., 0 kg N ha−1 and 100 kg N ha−1) and three water levels (i.e., rainfed, water deficit imposed between stem elongation and flowering and full irrigation) were randomly assigned to each plot. The water stress effects were investigated in another study [27]. In this study, different water treatments were considered to have the effect of enhancing plant status variability, as it is expected in actual agricultural fields.
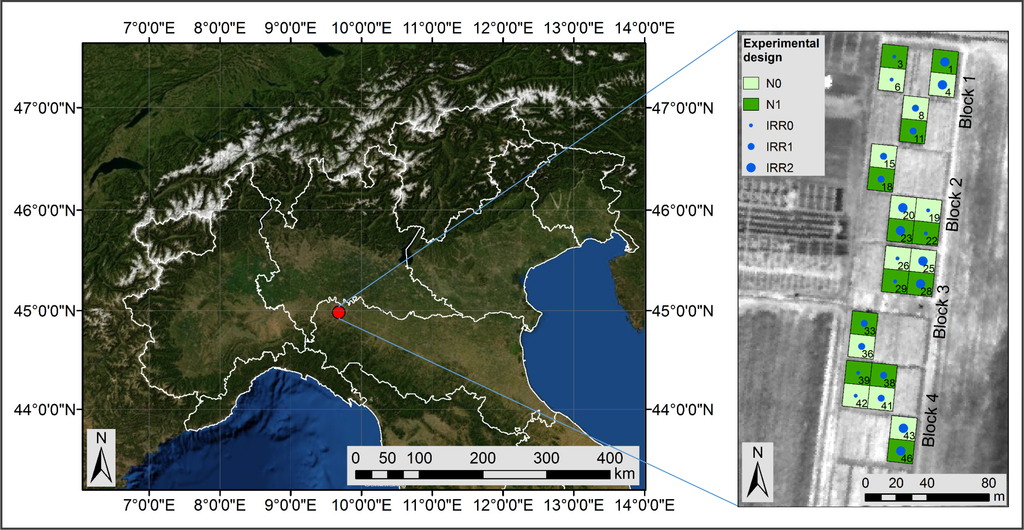
Figure 1. Experimental field location in Northern Italy and treatment scheme. Light green represents the not fertilised maize plots (N0) and dark green the plots treated with 100 kg N ha−1 (N1). The irrigation levels are shown as small circles in rainfed plots (IRR0), medium circles in water deficit plots (IRR1) and large circles in full irrigation plots (IRR2).The field was sown on 3 June 2010, and N fertiliser was applied manually twenty-one days after sowing. Other information concerning the experimental management plan is reported in Table 1.
Table 1. Field management plan.
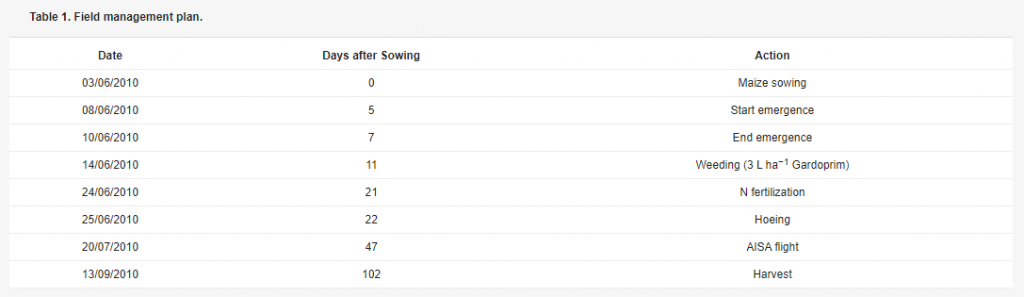
2.2. Field Data
A field campaign was conducted contemporary to the flight on the 20 July 2010. At the time of the flight plants were in their pre-flowering stem elongation stage [28] with an average of 10 leaves expanded.All measurements were taken in an area of 3 m × 3 m centered in the plots. Measurements were collected through destructive (i.e., actual N concentration (%Na), plant dry mass and grain production) and non-destructive (i.e., foliar measurements reported in Table 2 and Leaf Area Index (LAI)) methods. The micro-Kjeldahl method was used to measure the %Na from leaf samples collected immediately after the image acquisition from four plants per plot. Plant dry mass was measured immediately after the image acquisition (Wflight) in plot centers and at harvest (Wend). The %Na and the Wflight were used for the estimation of the NNI in the field (NNIfield).
Table 2. Non-destructive foliar measurements acquired in each plot center. Relative chlorophyll content (Cab), photosynthetic yield (ΔF/Fm′) and instantaneous CO2 assimilation (Ai) were acquired on the last fully expanded leaf. Each measure is the average of three acquisitions.

The non-destructive measurements were acquired on the last fully expanded leaf. Relative chlorophyll content (Cab) was measured with a SPAD-502 meter (Minolta, Tokyo, Japan) [29]. Instantaneous CO2 assimilation (Ai) was recorded with a CIRAS-1 (PP-Systems, Amesbury, MA, USA) and photosynthetic yield (ΔF/Fm′) with a Photosynthesis Yield Analyzer Mini-PAM (Walz, Effeltrich, Germany). ΔF/Fm′ represents the efficiency of electron transport by photosystem II (PSII) under steady-state conditions of actual irradiance. Higher ΔF/Fm′ values are typical of healthier leaves: ΔF is the difference between Fm′, the maximal fluorescence yield of the sample under environment illumination and Ft, the fluorescence yield under environment illumination measured before the saturation pulse [30]. LAI (Leaf Area Index) is the measure of the green area per soil unit area, and was estimated in each plot center using a linear ceptometer in the PAR (Photosynthetically Active Radiation) domain (SunScan Canopy Analysis System, Delta-T devices, Burwell, UK). LAI estimation with such a device requires the measurement of incident (direct and diffuse) and transmitted radiation. The incident radiation was measured from about 0.5 m above the top of the canopy in each plot. The direct to diffuse radiation ratio was then estimated by conducting a second incident radiation measurement after shadowing about 1/4 of the probe sensors (100 cm long) according to [31]. Seven LAI measurements were then conducted and averaged along a transect crossing two consecutive crop rows, by measuring the transmitted solar radiation at ground level and exploiting the SunScan internal software for LAI estimation [31]. For the LAI estimation from such measurements, leaf absorption in the PAR domain was assumed equal to 0.85, while the ellipsoidal leaf angle distribution parameter was set to 1.37 [32].
2.3. Nitrogen Nutrition Index
The NNIfield [6] was calculated with the destructive measurements conducted in plot centers contemporary to the flight. The NNI reported in Equation (1) is the ratio between the %Na and the %Nc as a percentage of dry mass. The %Na is the leaf actual N concentration measured in the field and the %Nc is the minimum N concentration necessary to achieve the maximum aboveground dry mass, expressed by Equation (2), where a and b are crop dependent constants and W (t ha−1) corresponds to the plant dry mass:NNI=%Na%NcNNI=%Na%Nc(1)%Nc=a×(W)−b%Nc=a×(W)−b(2)According to [33], the coefficients a and b were set equal to 3.40 and 0.37, respectively. NNI values close to 1 indicate plants not limited by N availability, values lower than 1 indicate N deficiency and values higher than 1 indicate that N accumulation occurs without an increase in crop biomass.
2.4. Hyperspectral Data Acquisition
A hyperspectral image was acquired at 12.37 UTC on the 20 July 2010 with the AISA Eagle sensor flown in the solar principal plane by the Italian Istituto Nazionale di Oceanografia e Geofisica Sperimentale (OGS). Acquisition parameters are given in Table 3.
Table 3. Sensor characteristics and spectral region covered. FWHM is the Full Width at Half Maximum, IFOV is the Instantaneous Field Of View.

The AISA image was georeferenced with CaliGeo software (Specim, Oulu, Finland) using data from the GPS/IMU unit onboard. The image was atmospherically corrected by the empirical line approach using ground reflectance spectra measured in field at the time of the flight [34] by means of a FieldSpec Pro portable spectroradiometer (ASD, Boulder, CO, USA). The empirical line approach is used to force image radiance (L) to match selected field reflectance spectra by means of a linear regression for each acquisition band. Two 6 m × 6 m calibration panels (white and black Odyssey material (Kayospruce, Fareham, UK)) were measured. Homogeneous targets with lambertian behaviour (two soils and one asphalt) were also measured to improve the correction accuracy [35]. Fitting accuracy between field spectral signatures and remotely sensed data was then evaluated in each band through the Root Mean Square Error (RMSE) and resulted in less than 1% of reflectance.
2.5. Vegetation Index Computation
The plot centers were located on the AISA image and mean reflectance values were extracted from 3 × 3 pixel areas (9 m2). Several narrowband vegetation indices were calculated. As reported in Table 4 [21,36–43], indices are divided into three categories on the basis of previous findings in literature: indices mainly related to N, to foliar pigments and to green biomass.
Table 4. Narrowband vegetation indices tested in this study. Rx is the reflectance at the wavelength x, expressed in nm. AISA band centers used for calculations are: 550.51 (R550), 669.55 (R670), 681.52 (R681.25), 700.72 (R700), 707.94 (R708.75), 719.96 (R720), 753.70 (R753.75) and 799.81 (R800).
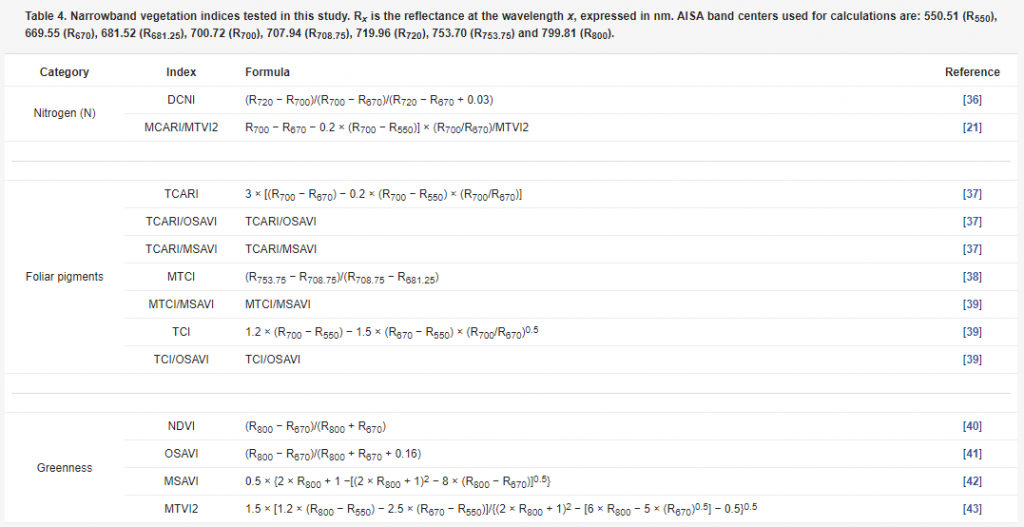
Two recent indices proposed for the estimation of N content, DCNI (Double-peak Canopy Nitrogen Index) and MCARI/MTVI2 (Modified Chlorophyll Absorption Ratio Index/Modified Triangular Vegetation Index 2), were tested. The following foliar pigment indices were considered: TCARI (Transformed Chlorophyll Absorption in Reflectance Index), MTCI (MERIS Terrestrial Chlorophyll Index), and TCI (Triangular Chlorophyll Index). In addition, NDVI (Normalised Difference Vegetation Index) and soil adjusted vegetation indices OSAVI (Optimised Soil Adjusted Vegetation Index), MSAVI (Modified Soil Adjusted Vegetation Index), and MTVI2 were tested as greenness indices. The combination of foliar pigment indices with soil adjusted vegetation indices was also tested as indicator of foliar pigments with the aim of normalising for differences in canopy structure and soil contribution.
2.6. NNI and Variable Rate N Fertilisation Maps
The regression analyses between field data and vegetation indices allowed the selection of the best indices for the estimation of the NNI input parameters (i.e., %Na and W).Once the NNI map was calculated, we proposed an empirical method to quantify the N deficit or surplus in the field (Nstatus) expressed as (g N m−2). For this purpose, the actual N content accumulated in the aboveground biomass (Nw) was calculated at pixel level as %Na × W (g m−2). The optimal Nw (Nw_opt) was calculated as the mean Nw value of pixels with NNI close to 1 (i.e., optimal NNI value). Nstatus was then calculated as the difference between Nw and Nw_opt. This allowed to map the Nstatus at the time of the flight overpass: positive values of Nstatus indicated areas characterised by N surplus, otherwise negative values of Nstatus indicated N deficit. The N deficit represents the amount of N to be prescribed in order to provide the optimal N content to improve the maize production.
2.7. Statistical Analyses
The statistical differences between biochemical and physiological parameters measured in N0 and N1 plots were tested through the Student t test. Relationships between field data and vegetation indices were evaluated by Ordinary Least Squares (OLS) regression analyses in order to determine best indices for %Na and Wflight estimation on the basis of the higher coefficient of determination (R2). Different fitting function models were tested (i.e., linear, power, logarithmic, and exponential).
3. Results
3.1. Field Data
The statistical difference between biochemical and physiological parameters measured in N0 and N1 plots was tested. As expected higher average values occurred in N1 plots, even though differences between treatments were not always significant. Results are reported in Table 5.
Table 5. Statistical analyses (t test) on fertilisation effect on field parameters measured in maize plot centres. Mean value, standard deviation and p value are reported. In case of statistical significance bold letters indicate different groups (p < 0.05). Analyses were performed on 4 blocks (24 plots), except for Ai (1 block, 6 plots).
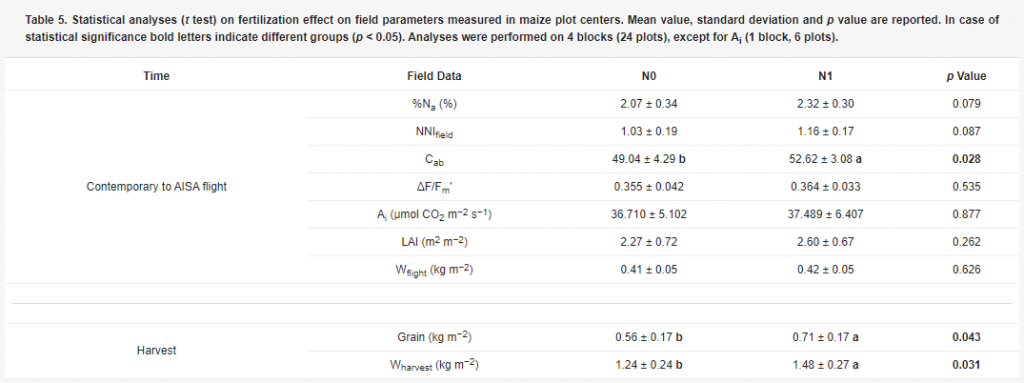
The only parameter affected by N supplied to the maize plots at the time of the flight was Cab (p = 0.028). Dry mass (Wflight) differences between fertilisations were in fact not significant, meaning that differences in N supplies did not affect plant development at this stage. Instead, some differences occurred at the end of the experiment in Wharvest (p = 0.031) and in grain production (p = 0.043).As expected, the known relationship between N and chlorophyll content [19,36] was confirmed in our data, since a statistically significant relationship (p < 0.05) was found between %Na and Cab (R2 = 0.56). However, contrary to Cab, significant differences between N treatments were not detected in %Na (p = 0.079) and NNIfield (p = 0.087).
3.2. Vegetation Indices Regressions
Regression analyses between field data and vegetation indices were performed to select the indices providing the best results in mapping %Na and W, which are the input parameters for the NNI computation. The coefficients of determination and the significance of the linear regression models are reported in Table 6; the R2 of power, logarithmic or exponential models is reported only when it was higher than the linear one.
Table 6. Regression analyses between vegetation indices and field data measured in the 24 plot centers: photosynthetic yield (ΔF/Fm′), actual N concentration (%Na), relative chlorophyll content (Cab), Leaf Area Index (LAI) and dry mass (Wflight) measured during the flight. Coefficient of determination (R2) and significance (***p < 0.001, **p < 0.010, *p < 0.05) are reported. Linear models were used if not specified with abbreviations in parenthesis: power (pw) or logarithmic (ln).
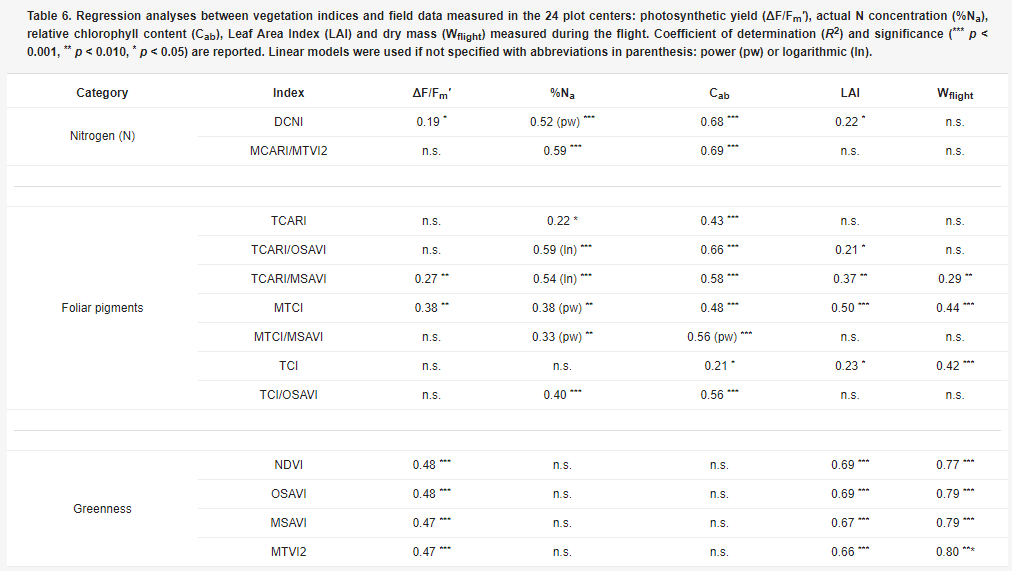
Combined chlorophyll indices (i.e., MCARI/MTVI2, TCARI/OSAVI, TCARI/MSAVI, TCI/OSAVI) were better related with Cab and %Na than traditional chlorophyll vegetation indices, due to the minimisation of structural effects and soil contributions. The MCARI/MTVI2 showed the best performances in estimating both Cab (R2 = 0.69) and %Na (R2 = 0.59) while it was not significantly related to LAI. This suggested that MCARI/MTVI2 was the most suitable for %Na detection since it was not affected by canopy structure. The linear function obtained is reported in Figure 2a. All the greenness indices were well related to Wflight (R2 > 0.70). Soil adjusted indices (OSAVI, MSAVI, and MTVI2) performed slightly better than the traditional NDVI, since they were able to minimise the soil background effects on reflectance in case of low fractional cover. Among these the MTVI2 (R2 = 0.80) was selected for the dry mass estimation (Figure 2b).
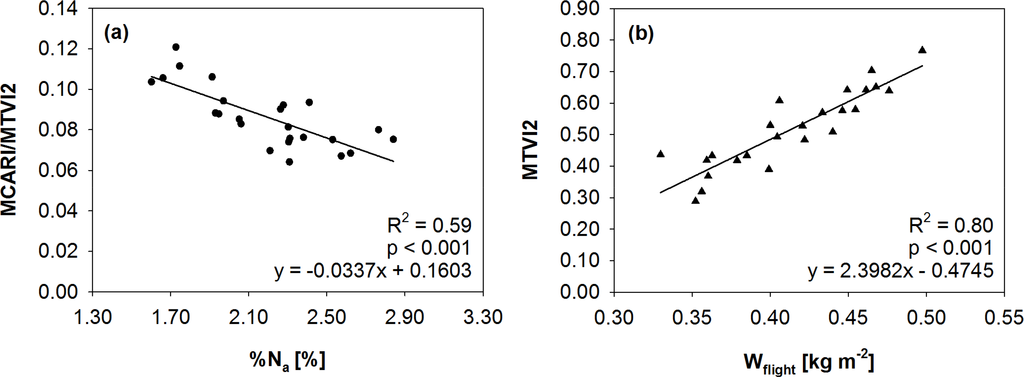
Figure 2. Linear regressions between field data and vegetation indices. (a) %Na and MCARI/MTVI2 (R2 = 0.59). (b) Wflight and MTVI2 (R2 = 0.80).
3.3. NNI and Variable Rate N Fertilisation Maps
The NNI map in Figure 3a was obtained through the combination of the input parameters (Equations (1) and (2)) estimated remotely with the relationships in Figure 2. The NNI range was divided in five classes represented with colors from red (low NNI values) to dark green (high NNI values) centered around the optimal NNI value (NNI = 1). As reported in Table 7, pixels showing N deficit (NNI ≤ 0.9) represented the 23% of the entire field, pixels with optimal N supply (0.9 < NNI ≤ 1.1) represented the 23% while pixels with N surplus (NNI > 1.1) represented the 54%.
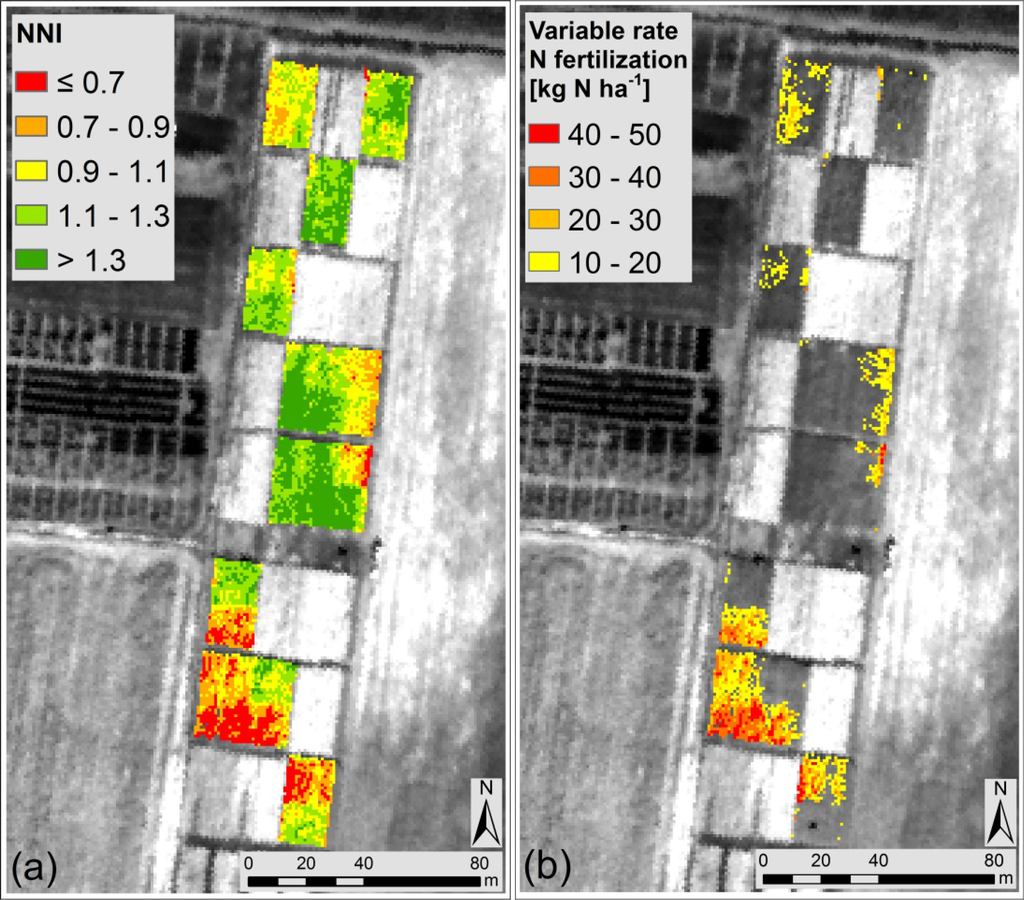
Figure 3. Maps obtained over the maize experimental field. (a) NNI (Nitrogen Nutrition Index) map obtained from remotely sensed data. Classes were defined around the optimal NNI value (NNI = 1). (b) Variable rate N fertilisation map (kg N ha−1) on the basis of the Nstatus value in each pixel. The suggested rate is shown only for pixels belonging to N deficient areas (i.e., NNI ≤ 0.9).
Table 7. NNI (Nitrogen Nutrition Index) classes, corresponding area, description of the area with respect to the Nstatus, actual N content accumulated in the aboveground biomass (Nw) and Nstatus mean values (g N m−2).
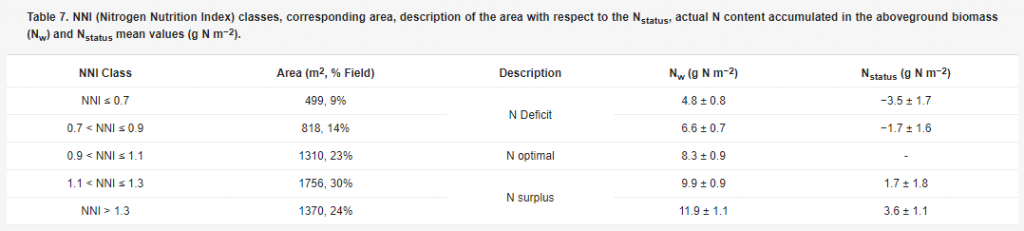
A good agreement (R2 = 0.70, p < 0.001) was found between the mean NNI estimated for each parcel from remote sensing and the NNIfield, showing that the remote estimation of NNI is coherent with the traditional one measured in the field. The suitability of the NNI map to detect the N status was also evaluated analysing the statistical difference between plots treated with different N amounts: the mean NNI value in N0 plots (NNI = 0.99 ± 0.23) was statistically different from the mean NNI value in N1 (NNI = 1.21 ± 0.17) (p = 0.016). It was observed that in general the field was not in a critical N deficit condition since mean values in N0 and N1 plots were both in the range of optimal N supply (i.e., NNI close to 1).The optimal N content (Nw_opt) was computed as the mean Nw from the optimal NNI pixels (0.9 < NNI ≤ 1.1) and was found equal to 8.3 g N m−2. This value was used together with the Nw in each pixel to estimate the Nstatus. Results are reported in Table 7 for each NNI class. The Nstatus calculated at pixel level was converted in variable rate N fertilisation (kg N ha−1) as reported in Figure 3b. The variable rate N fertilisation quantity should allow to obtain a Nstatus equal to zero in each N deficient pixel, in order to reproduce the Nstatus typical of optimal areas. The suggested rate is shown only for pixels belonging to N deficient areas (i.e., NNI ≤ 0.9).
4. Discussions
As observed from the field data, the availability of different N amounts did not lead to prominent visual or structural effects at the time of the image acquisition, allowing the investigation of a method for N deficiency detection in an early phase. In fact, at the time of the flight, statistically significant differences related to N supplies were observed only for Cab while the canopy structure parameters were unaffected. Remote sensing techniques have been already used to monitor leaf chlorophyll and N concentration in agriculture in the context of precision farming practices: several studies conducted on maize crops report leaf chlorophyll concentration [37,39] as well as leaf N concentration maps [36,44] reproducing the spatial patterns related to different N supplies and soil conditions. Other studies focused on the estimation of crop density from aerial imagery [45,46] and from sensors onboard agricultural machineries to drive fertilisation rates in real time [47,48]. Recently [9] showed that spectral data collected on wheat with a tractor mounted field spectrometer were related to the NNI measured in the field. These spectral systems analyse the areas surrounding the tractor and may be a useful tool in small or medium-sized fields. However, when the field extension is considerably larger, aerial tools may become necessary. Although the attention toward precision farming techniques is increasing, studies providing methods to obtain variable rate N fertilisation maps based on remote sensing data are still limited. Among remote sensing techniques it is worth mentioning the recent development of UAVs (Unmanned Aerial Vehicles) in agricultural applications allowing the collection of multispectral and hyperspectral imagery at sub-metric spatial resolution [49–52].The possibility to map the NNI from airborne hyperspectral data found limited application so far; therefore, this study represents an innovative attempt to produce a variable rate N fertilisation map. It should be noted that the integration of radiometric data and crop models addressed in other studies [53] would constitute an approach able to consider also climate and soil conditions. Our methodology allowed to merge the information about actual N concentration and dry mass identifying the areas where both parameters were low: a site-specific management was suggested over the 23% of the field, instead of an extensive fertilisation. It must be noted that the proposed pragmatic approach to determine the Nstatus requires that a number of pixels with NNI close to 1 are present in the scene. Since no recovery fertilisation occurred after the flight, the deficiencies remained until the end of the growing season as shown by the differences in grain production. Even though a good agreement between NNI map and treatments was generally found, we observed some NNI values higher than 1 in few N0 plots, indicating N surplus: this could be due to nutrient residuals from previous agricultural practices. Furthermore, some low NNI values were observed in correspondence of water stressed plots (rainfed plots) even if supplied with N. This evidenced that in case water was a limiting factor plants were not able to use efficiently the supplied N and this affected the regular plant development. Remotely sensed indicators of water stress (i.e., canopy temperature, passive fluorescence, and PRI) able to highlight conditions of critical water supply [27,54] might be integrated with the NNI to produce N and water variable rate maps. In this way, knowing the amount of fertiliser administered to each parcel and the current water status, it would be possible to evaluate if the observed N deficit is due to a lack of fertilisation or irrigation. The Nstatus was quantified with reference to the average Nw shown by pixels in optimal conditions according to the NNI map (0.9 < NNI ≤ 1.1). The deficit found in the plant Nstatus was used to prescribe the amount of N that should be applied to the soil by the farmer; further investigation would be needed in order to predict the N availability for plants when fertilisers are applied to the soil, considering the losses mainly due to water leakage. Moreover, further studies should address a higher number of fertilisation supplies to better evaluate the proposed method effectiveness.
5. Conclusions
This study proved that airborne hyperspectral imagery can be used to detect N deficient areas in maize crops. The parameters of interest for the production of the NNI (Nitrogen Nutrition Index) map (i.e., the leaf actual N concentration (%Na) and the dry mass (Wflight)) were successfully estimated with indices MCARI/MTVI2 (R2 = 0.69) and MTVI2 (R2 = 0.80), respectively. The obtained map constitutes an innovative attempt to calculate the NNI from airborne data over an entire field and allowed to distinguish areas characterised by different N availability (i.e., deficit, optimal and surplus). The good agreement between the NNI calculated from remote sensing and the NNI from field measurement (R2 = 0.70) supports the use of aerial data instead of traditional time consuming measurements. The NNI map represented a crucial step for the production of a variable rate N fertilisation map to be used for a rational management of the field, based on the comparison between the N accumulated in the aboveground biomass in each pixel (Nw) and the mean value of N found in optimal areas calculated through the NNI map (Nw_opt). The difference between Nw and Nw_opt (Nstatus) allowed to identify N deficit areas in correspondence of pixels where the Nstatus assumed a negative value. Only the 23% of the field was identified as N deficient and the maximum suggested fertilisation rate was equal to 50 kg N ha−1. It is worth noting that the presence of vegetation in optimal conditions in the scene is necessary to compute Nw_opt and consequently Nstatus. This makes the method hardly applicable in field characterised by a widespread N deficiency where N optimal areas cannot be identified. The method presented in this study allowed to define the minimum amount of N to apply without decreasing crop production and at the same time avoiding excessive fertilisation in order to guarantee a proper management of the environmental resources in agricultural practices. Furthermore, the availability of measurements repeated over time could offer a valuable tool for a more sustainable field management during the growing season.
Acknowledgments
This research was funded by Axìa (CRUI-Nestlé project for research and development). Field campaigns were conducted in the frame of EUFAR (European Facility For Airborne Research) Transnational Access project. The authors gratefully acknowledge S. Cogliati, A. Marchesi, B. Di Mauro, T. Julitta, M. Ferretti (DISAT, University of Milano-Bicocca), M. Bergonti (Università Cattolica di Piacenza), M. Musanti (CNR-IREA Milano), F. Pinto (Forschungszentrum Jülich) for their participation to the field campaign.
Author Contributions
All authors participated to the measurement collection that was part of an intensive field campaign. Chiara Cilia wrote the manuscript supported in both data interpretation and manuscript writing by Cinzia Panigada and Micol Rossini. Cinzia Panigada was the technical coordinator of the campaign and planned the experimental design with Michele Meroni. A significant contribution to the field data interpretation was also provided by Michele Meroni, Lorenzo Busetto and Valentina Picchi. Mirco Boschetti performed the image atmospheric correction and the related radiometric field measurements. Stefano Amaducci was responsible for the experimental field management and Roberto Colombo was the project lead scientist.
Conflicts of Interest
The authors declare no conflict of interest.
References
- Mosier, A.; Kroeze, C.; Nevison, C.; Oenema, O.; Seitzinger, S.; van Cleemput, O. Closing the global N(2)O budget: Nitrous oxide emissions through the agricultural nitrogen cycle—OECD/IPCC/IEA phase II development of IPCC guidelines for national greenhouse gas inventory methodology. Nutr. Cycl. Agroecosyst 1998, 52, 225–248. [Google Scholar]
- Sehy, U.; Ruser, R.; Munch, J.C. Nitrous oxide fluxes from maize fields: Relationship to yield, site-specific fertilisation, and soil conditions. Agric. Ecosyst. Environ 2003, 99, 97–111. [Google Scholar]
- Schroder, J.J.; Neeteson, J.J.; Oenema, O.; Struik, P.C. Does the crop or the soil indicate how to save nitrogen in maize production? Reviewing the state of the art. Field Crop. Res 2000, 66, 151–164. [Google Scholar]
- Justes, E.; Mary, B.; Meynard, J.M.; Machet, J.M.; Thelierhuche, L. Determination of a critical nitrogen dilution curve for winter-wheat crops. Ann. Bot 1994, 74, 397–407. [Google Scholar]
- Greenwood, D.J.; Lemaire, G.; Gosse, G.; Cruz, P.; Draycott, A.; Neeteson, J.J. Decline in percentage N of C3 and C4 crops with increasing plant mass. Ann. Bot 1990, 66, 425–436. [Google Scholar]
- Lemaire, G.; Jeuffroy, M.H.; Gastal, F. Diagnosis tool for plant and crop N status in vegetative stage theory and practices for crop N management. Eur. J. Agron 2008, 28, 614–624. [Google Scholar]
- Lemaire, G.; Gastal, F. Quantifying responses of crop species to N nutrition deficiency: Improving N use efficiency. In Crop Physiology: Applications for Genetic Improvement and Agronomy; Sadras, V.O., Calderini, D.F., Eds.; Academic Press: San Diego, CA, USA, 2008; pp. 171–211. [Google Scholar]
- Vouillot, M.O.; Huet, P.; Boissard, P. Early detection of N deficiency in a wheat crop using physiological and radiometric methods. Agronomie 1998, 18, 117–130. [Google Scholar]
- Mistele, B.; Schmidhalter, U. Estimating the nitrogen nutrition index using spectral canopy reflectance measurements. Eur. J. Agron 2008, 29, 184–190. [Google Scholar]
- Houles, V.; Guerif, M.; Mary, B. Elaboration of a nitrogen nutrition indicator for winter wheat based on leaf area index and chlorophyll content for making nitrogen recommendations. Eur. J. Agron 2007, 27, 1–11. [Google Scholar]
- Panigada, C.; Rossini, M.; Busetto, L.; Meroni, M.; Fava, F.; Colombo, R. Chlorophyll concentration mapping with MIVIS data to assess crown discoloration in the Ticino Park oak forest. Int. J. Remote Sens 2010, 31, 3307–3332. [Google Scholar]
- Blackburn, A. Hyperspectral remote sensing of plant pigments. Comp. Biochem. Physiol. A Mol. Integr. Physiol 2006, 143, S147–S147. [Google Scholar]
- Rossini, M.; Panigada, C.; Meroni, M.; Colombo, R. Assessment of oak forest condition based on leaf biochemical variables and chlorophyll fluorescence. Tree Physiol 2006, 26, 1487–1496. [Google Scholar]
- Yu, K.; Leufen, G.; Hunsche, M.; Noga, G.; Chen, X.; Bareth, G. Investigation of leaf diseases and estimation of chlorophyll concentration in seven barley varieties using fluorescence and hyperspectral indices. Remote Sens 2014, 6, 64–86. [Google Scholar]
- Daughtry, C.S.T.; Walthall, C.L.; Kim, M.S.; de Colstoun, E.B.; McMurtrey, J.E. Estimating corn leaf chlorophyll concentration from leaf and canopy reflectance. Remote Sens. Environ 2000, 74, 229–239. [Google Scholar]
- Thenkabail, P.S.; Enclona, E.A.; Ashton, M.S.; van der Meer, B. Accuracy assessments of hyperspectral waveband performance for vegetation analysis applications. Remote Sens. Environ 2004, 91, 354–376. [Google Scholar]
- Evans, J.R. Photosynthesis and nitrogen relationships in leaves of C3 plants. Oecologia 1989, 78, 9–19. [Google Scholar]
- Evans, J.R. Nitrogen and photosynthesis in the flag leaf of wheat (Triticum-aestivum L.). Plant Physiol 1983, 72, 297–302. [Google Scholar]
- Schlemmer, M.; Gitelson, A.; Schepers, J.; Ferguson, R.; Peng, Y.; Shanahan, J.; Rundquist, D. Remote estimation of nitrogen and chlorophyll contents in maize at leaf and canopy levels. Int. J. Appl. Earth Obs. Geoinf 2013, 25, 47–54. [Google Scholar]
- Stroppiana, D.; Boschetti, M.; Brivio, P.A.; Bocchi, S. Plant nitrogen concentration in paddy rice from field canopy hyperspectral radiometry. Field Crops Res 2009, 111, 119–129. [Google Scholar]
- Eitel, J.U.H.; Long, D.S.; Gessler, P.E.; Smith, A.M.S. Using in-situ measurements to evaluate the new RapidEye (TM) satellite series for prediction of wheat nitrogen status. Int. J. Remote Sens 2007, 28, 4183–4190. [Google Scholar]
- Zhang, J.H.; Wang, K.; Bailey, J.S.; Wang, R.C. Predicting nitrogen status of rice using multispectral data at canopy scale. Pedosphere 2006, 16, 108–117. [Google Scholar]
- Graeff, S.; Claupein, W. Quantifying nitrogen status of corn (Zea mays L.) in the field by reflectance measurements. Eur. J. Agron 2003, 19, 611–618. [Google Scholar]
- Yoder, B.J.; Pettigrewcrosby, R.E. Predicting nitrogen and chlorophyll content and concentrations from reflectance spectra (400–2500 nm) at leaf and canopy scales. Remote Sens. Environ 1995, 53, 199–211. [Google Scholar]
- Psomas, A.; Kneubuhler, M.; Huber, S.; Itten, K.; Zimmermann, N.E. Hyperspectral remote sensing for estimating aboveground biomass and for exploring species richness patterns of grassland habitats. Int. J. Remote Sens 2011, 32, 9007–9031. [Google Scholar]
- Hatfield, J.L.; Prueger, J.H. Value of using different vegetative indices to quantify agricultural crop characteristics at different growth stages under varying management practices. Remote Sens 2010, 2, 562–578. [Google Scholar]
- Panigada, C.; Rossini, M.; Meroni, M.; Cilia, C.; Busetto, L.; Amaducci, S.; Boschetti, M.; Cogliati, S.; Picchi, V.; Pinto, F.; et al. Fluorescence, PRI and canopy temperature for water stress detection in cereal crops. Int. J. Appl.Earth Obs. Geoinf 2014, 30, 167–178. [Google Scholar]
- Lancashire, P.D.; Bleiholder, H.; Langeluddecke, P.; Stauss, R.; van den Boom, T.; Weber, E.; Witzenberger, A. A uniform decimal code for growth stages of crops and weeds. Ann. Appl. Biol 1991, 119, 561–601. [Google Scholar]
- Markwell, J.; Osterman, J.C.; Mitchell, J.L. Calibration of the Minolta SPAD-502 leaf chlorophyll meter. Photosynth. Res 1995, 46, 467–472. [Google Scholar]
- Genty, B.; Briantais, J.M.; Baker, N.R. The relationship between the quantum yield of photosynthetic electron transport and quenching of chlorophyll fluorescence. Biochim. Biophys. Acta 1989, 990, 87–92. [Google Scholar]
- Webb, N.; Nichol, C.; Wood, J.; Potter, E. User Manual for the SunScan Canopy Analysis System, (2.0 Version); Delta-T Devices Ltd.: Cambridge, UK, 2008; p. 83. [Google Scholar]
- Van Evert, F.K.; Campbell, G.S. CropSyst: A collection of object-oriented simulation models of agricultural systems. Agron. J 1994, 86, 325–331. [Google Scholar]
- Plenet, D.; Lemaire, G. Relationships between dynamics of nitrogen uptake and dry matter accumulation in maize crops. Determination of critical N concentration. Plant Soil 1999, 216, 65–82. [Google Scholar]
- Smith, G.M.; Milton, E.J. The use of the empirical line method to calibrate remotely sensed data to reflectance. Int. J. Remote Sens 1999, 20, 2653–2662. [Google Scholar]
- Baugh, W.M.; Groeneveld, D.P. Empirical proof of the empirical line. Int. J. Remote Sens 2008, 29, 665–672. [Google Scholar]
- Chen, P.; Haboudane, D.; Tremblay, N.; Wang, J.; Vigneault, P.; Li, B. New spectral indicator assessing the efficiency of crop nitrogen treatment in corn and wheat. Remote Sens. Environ 2010, 114, 1987–1997. [Google Scholar]
- Haboudane, D.; Miller, J.R.; Tremblay, N.; Zarco-Tejada, P.J.; Dextraze, L. Integrated narrow-band vegetation indices for prediction of crop chlorophyll content for application to precision agriculture. Remote Sens. Environ 2002, 81, 416–426. [Google Scholar]
- Dash, J.; Curran, P.J. The MERIS terrestrial chlorophyll index. Int. J. Remote Sens 2004, 25, 5403–5413. [Google Scholar]
- Haboudane, D.; Tremblay, N.; Miller, J.R.; Vigneault, P. Remote estimation of crop chlorophyll content using spectral indices derived from hyperspectral data. IEEE Trans. Geosci. Remote Sens 2008, 46, 423–437. [Google Scholar]
- Rouse, J.W.; Haas, R.H.; Schell, J.A.; Deering, D.W.; Harlan, J.C. Monitoring the Vernal Advancements and Retro Gradation of Natural Vegetation; NASA/GSFC Final Report. Greenbelt, MD, USA, 1974; p. 371. Available online: http://ntrs.nasa.gov/archive/nasa/casi.ntrs.nasa.gov/19740004927.pdf (accessed on 19 March 2014).
- Rondeaux, G.; Steven, M.; Baret, F. Optimisation of soil-adjusted vegetation indices. Remote Sens. Environ 1996, 55, 95–107. [Google Scholar]
- Qi, J.; Chehbouni, A.; Huete, A.R.; Kerr, Y.H.; Sorooshian, S. A modified soil adjusted vegetation index. Remote Sens. Environ 1994, 48, 119–126. [Google Scholar]
- Haboudane, D.; Miller, J.R.; Pattey, E.; Zarco-Tejada, P.J.; Strachan, I.B. Hyperspectral vegetation indices and novel algorithms for predicting green LAI of crop canopies: Modeling and validation in the context of precision agriculture. Remote Sens. Environ 2004, 90, 337–352. [Google Scholar]
- Boegh, E.; Soegaard, H.; Broge, N.; Hasager, C.B.; Jensen, N.O.; Schelde, K.; Thomsen, A. Airborne multispectral data for quantifying leaf area index, nitrogen concentration, and photosynthetic efficiency in agriculture. Remote Sens. Environ 2002, 81, 179–193. [Google Scholar]
- Grenzdorffer, G.J. Investigations on the use of airborne remote sensing for variable rate treatments of fungicides, growth regulators and N-fertilisation. Precision Agric. 2003, 241–246. [Google Scholar]
- Johnson, L.F.; Bosch, D.F.; Williams, D.C.; Lobitz, B.M. Remote sensing of vineyard management zones: Implications for wine quality. Appl. Eng. Agric 2001, 17, 557–560. [Google Scholar]
- Schwab, G.J.; Pena-Yewtukhiw, E.M.; Wendroth, O.; Murdock, L.W.; Stombaugh, T. Wheat yield population response to variable rate N fertilisation strategies using active NDVI sensors. Precision Agric 2005, 5, 235–242. [Google Scholar]
- Shaver, T.; Khosla, R.; Westfall, D. Evaluation of two crop canopy sensors for nitrogen recommendations in irrigated maize. J. Plant Nutr 2014, 37, 406–419. [Google Scholar]
- Calderón, R.; Montes-Borrego, M.; Landa, B.B.; Navas-Cortés, J.A.; Zarco-Tejada, P.J. Detection of downy mildew of opium poppy using high-resolution multi-spectral and thermal imagery acquired with an unmanned aerial vehicle. Precision Agric 2014. [Google Scholar] [CrossRef]
- Quemada, M.; Gabriel, J.L.; Zarco-Tejada, P. Airborne hyperspectral images and ground-level optical sensors as assessment tools for maize nitrogen fertilisation. Remote Sens 2014, 6, 2940–2962. [Google Scholar]
- Zarco-Tejada, P.J.; Gonzalez-Dugo, V.; Berni, J.A.J. Fluorescence, temperature and narrow-band indices acquired from a UAV platform for water stress detection using a micro-hyperspectral imager and a thermal camera. Remote Sens. Environ 2012, 117, 322–337. [Google Scholar]
- Berni, J.A.J.; Zarco-Tejada, P.J.; Suarez, L.; Fereres, E. Thermal and narrowband multispectral remote sensing for vegetation monitoring from an unmanned aerial vehicle. IEEE Trans. Geosci. Remote Sens 2009, 47, 722–738. [Google Scholar]
- Baret, F.; Houles, V.; Guerif, M. Quantification of plant stress using remote sensing observations and crop models: The case of nitrogen management. J. Exp. Bot 2007, 58, 869–880. [Google Scholar]
- Rossini, M.; Fava, F.; Cogliati, S.; Meroni, M.; Marchesi, A.; Panigada, C.; Giardino, C.; Busetto, L.; Migliavacca, M.; Amaducci, S.; et al. Assessing canopy PRI from airborne imagery to map water stress in maize. ISPRS J. Photogramm. Remote Sens 2013, 86, 168–177. [Google Scholar]
© 2014 by the authors; licensee MDPI, Basel, Switzerland This article is an open access article distributed under the terms and conditions of the Creative Commons Attribution license (http://creativecommons.org/licenses/by/3.0/).
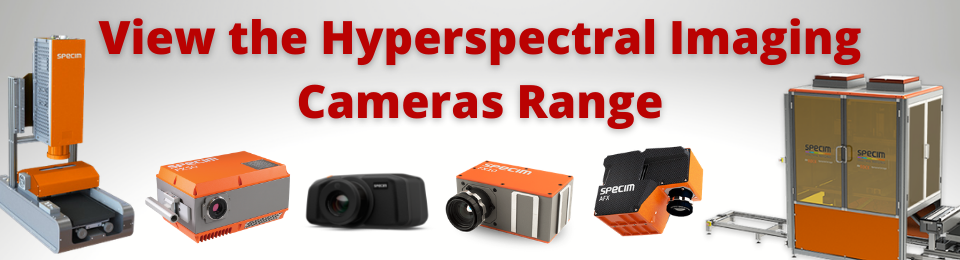